A few years ago, traditional chatbots were all the rage, and consequently, Google, Microsoft, and many others offered frameworks that can help you build chatbots.
They are all built around setting up a decision tree to steer the conversation to an endpoint and some natural language processing (NLP) capabilities. The decision tree represents the logical flow of the interaction between the user and the bot.
An order-taking chatbot in a pizzeria could, for example, first ask you what kind of pizza you want and present you with the binary choice of vegetarian or non-vegetarian. After your first choice, you’ll be presented with the next step until you finish your order. NLP capabilities can be used to help understand written text input from the user.
Decision tree systems have a long history of being used to methodically come to conclusions in various scenarios, but they are falling out of fashion. Let’s examine the reasons why.
When traditional chatbots work
Before diving into why people are abandoning the decision tree approach, let us first look at some cases where it can work:
- In medical emergencies (911 calls), the call-taker is trained to go through a predefined decision tree in order to arrive at the fastest possible conclusion regarding the patient’s condition. This setting is time-critical and highly emotional, so a formalized and highly optimized schema makes a lot of sense.
- Simple exchanges with our bank or insurance company, such as checking a balance or reporting less complete insurance claims, can be handled well with a decision tree approach.
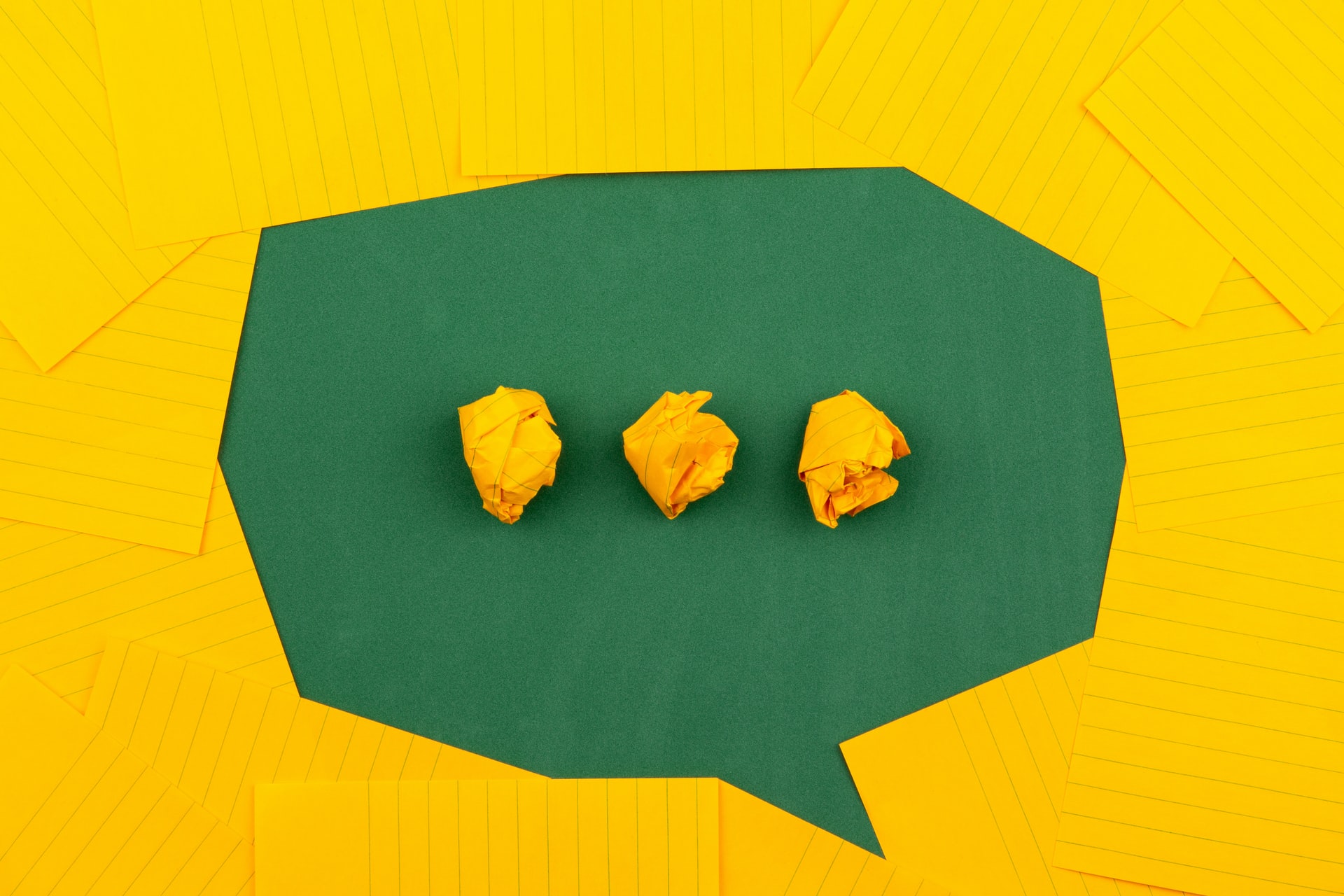
When traditional chatbots don’t work
Now to some of the reasons why the chatbot approach might be falling out of fashion:
- Complexity. Often the problems that need to be tackled are too complex to be represented by a mere decision tree.
- Laborious setup. It takes a lot of manual work to set up the best logical flow for the decision tree, and the solution could be vulnerable to sudden changes in circumstances.
- Lack of user-friendliness. Many users just want to get their questions answered and are not really interested in a long back-and-forth exchange to get the answer.
- Technological advances. AI technology, and more specifically deep learning-based NLP, has advanced so much in the last few years that we no longer need the chatbot approach. For example, the Google Duplex AI makes reservations by having a natural conversation with the restaurant. During the conversation, the AI negotiates the booking and makes sure that the call-taker has understood your intentions without the need for an explicit decision tree.
Traditional chatbots can be a convenient way to solve very specific problems requiring simple solutions. However, as we’ve seen above, they have their limitations.
Find out how Raffle Chat solves the problems that traditional chatbots can't - and how it can instantly upgrade your website experience.